Promoting Data Science for Accessibility with Publicly Available Datasets
- Mayanka
- Jan 27, 2018
- 5 min read
Supervised By: Dr. Hernisa Kacorri
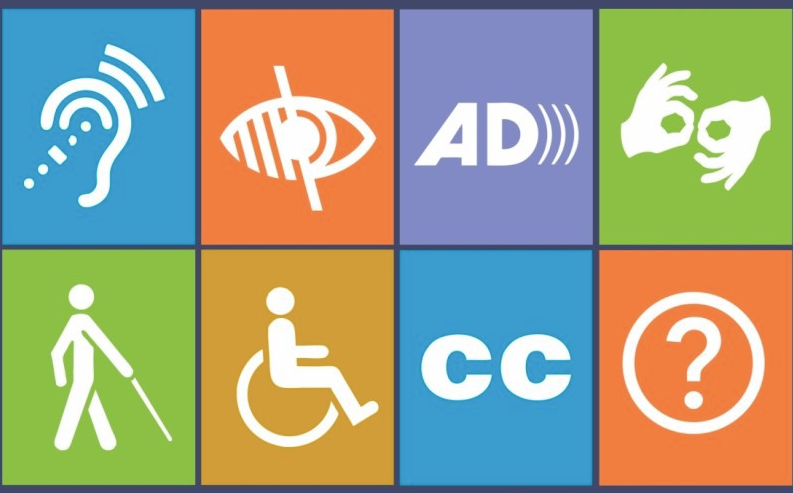
Motivation
Technology holds great promises for improving the quality of life for people with disabilities. With the explosion of machine learning and artificial intelligence (AI), several assistive technologies have emerged in the recent few years that enhance life experiences for people with disabilities such as predictive text-entry and brain computer interfaces [2], sign language synthesis and recognition [4], automatic object recognition [7], and indoor localization [3], to mention a few. Much of the recent progress in data-driven solutions is due to the availability of large datasets. Knowing the importance and cost of collecting and annotating datasets, researchers often make their resources publicly available. Public datasets has often served as a mean of attracting, nurturing, and challenging data scientists to work on specific problems, e.g., by creating problem-specific data science competitions. However, this
approach has seen limited use in the field of accessibility.
Goal of this proposal
We aim to promote data science for accessibility by increasing awareness on
challenges of accessing datasets from users with disabilities and creating a repository with currently available resources. To achieve this goal we propose to carry the following activities:
Collect and analyze public accessibility datasets on web.
Conduct surveys and interviews with students and trained data scientists on their potential interest in working on data-driven accessibility problems.
Create a repository of currently available accessibility datasets.
Prior Work
Beyond sharing resources and attracting new researchers in a new problem, public datasets
are often used for benchmarking purposes. For example, ImageNet [5], a dataset with millions of annotated images, is an established benchmark that helped researchers test and report the accuracy of their object classification approaches and thus advanced the computer vision field. For this reasons, when a new data-driven research area emerges, researchers typically survey and analyze related datasets that have been publicly released (e.g., [6]). However, to our knowledge no prior work has surveyed and analyzed existing
datasets in the field of accessibility.
Preliminary Work
During the Fall 2017 semester we have manually examined existing online resources
with public datasets such as Kaggle [1], ACM, and IEEE to find accessibility datasets that are publicly available. We found that the release of datasets has been recently adopted by very few accessibility researchers, which tend to create unique links to these resources within their publications. Therefore, these resources tend to be spread throughout the web with no single repository referring to them. Many search strategies were unsuccessful for recovering these available resources leaving the manual inspection of individual papers as a
necessary step. This preliminary work emphasized the challenges faced by researchers that are new to this field in finding and accessing accessibility datasets, which could hinder data-driven research in this field.
Proposed Activities
As part of our preliminary work, we searched for datasets available at Kaggle. Out of the 8000 available datasets, only 20 were somehow related to people with disabilities – typically related to healthcare research and rarely to data-driven assistive technologies. This reinforced our belief that a detailed analysis needs to be carried out in order to unveil the challenges faced by researchers and data scientists interested in AI and accessibility problems.
Analyzing the collection of accessibility datasets: We will complete our collection of datasets by expanding to other venues beyond resources such as Kaggle. We found that accessibility research has been spread across many venues such as ACM, IEEE, LREC, ISCA, and ACL. Since these venues do not typically provide a database or a search engine for the available datasets we will use the search strategies that we deployed in our preliminary work such as: search by keywords (e.g., disability, impairment, accessibility, dataset, blind, visual impairment); search by conference (e.g., review papers from the last 10 years of ASSETS, the main ACM accessibility venue); search by authors that have previously released a dataset; and others. For each dataset we collect information on the user group and type of data. While the initial collection of dataset is almost complete, we will perform a descriptive analysis on our collection to inform the community on current trends for data sharing and compare it to similar collections in other fields.
Surveys and interviews: Going forward we want to conduct short surveys and interviews with few fellow data scientists working in accessibility. This will help us gain better insights into the need for shared resources in this field as well as the challenges researchers face in releasing accessibility datasets.. Moreover, we will approach data science communities and venues in the DC and surrounding regions for interviews. We aim to gain insights into potential interest and challenges in working with accessibility datasets as well as initial
feedback on our planned repository.
Repository: The last phase of this study will involve creating and launching an interactive web page with information on the collection of accessibility datasets. The goal of this page is to provide a public platform where people can find and share accessibility datasets. We will iteratively obtain feedback on our interface from fellow students at the iSchool interested in data science for good.
Outcomes and Impact
ndependence and quality of life. Our analysis of the dataset collection together with the findings from the surveys and interviews will ber data scientists across the world to find, share, and promote datasets and AI problems in the field of accessibility – ultimately benefiting people with disabilities by improving their independence and quality of life. Our analysis of the dataset collection together with the findings from the surveys and interviews will be submitted to the ACM SIGACCESS Conference on Computers and Accessibility (ASSETS 2018) as research paper. We anticipate that the sharing of the difficulties we faced while collecting the dataset and the insights from the analyses will benefit future researchers in the field.
References
[1] Kaggle. https: // www. kaggle. com/ . Accessed: 2018-01-10.
[2] Andrew Fowler, Brian Roark, Umut Orhan, Deniz Erdogmus, and Melanie Fried-Oken. Improved inference and autotyping in eeg-based bci typing systems. Proceedings of the 15th International ACM SIGACCESS Conference on Computers and Accessibility, page 15, 2013.
[3] Cole Gleason, Anhong Guo, Gierad Laput, Kris Kitani, and Jeffrey P Bigham. Vizmap: Accessible visual information through crowdsourced map reconstruction. Proceedings of the 18th International ACM SIGACCESS Conference on Computers and Accessibility, pages 273–274, 2016.
[4] Hernisa Kacorri and Matt Huenerfauth. Continuous profile models in asl syntactic facial expression synthesis. ACL (1), 2016.
[5] Olga Russakovsky, Jia Deng, Hao Su, Jonathan Krause, Sanjeev Satheesh, Sean Ma, Zhiheng Huang, Andrej Karpathy, Aditya Khosla, Michael Bernstein, et al. Imagenet large scale visual recognition challenge. International Journal of Computer Vision, 115(3):211–252, 2015.
[6] Iulian Vlad Serban, Ryan Lowe, Peter Henderson, Laurent Charlin, and Joelle Pineau. A survey of available corpora for building data-driven dialogue systems. arXiv preprint arXiv:1512.05742, 2015.
[7] Yu Zhong, Pierre J Garrigues, and Jeffrey P Bigham. Real time object scanning using a mobile phone and cloud based visual search engine. Proceedings of the 15th International ACM SIGACCESS Conference on Computers and Accessibility, page 20, 2013.
Comentarios